When Was AI Invented? A Comprehensive History
Artificial Intelligence (AI) has become a cornerstone of the modern digital experience. From chatbots and recommendation systems to complex automation, AI impacts nearly every facet of our lives. However, the journey from theoretical concept to integral technology wasn’t straightforward. In this article, we’ll explore the history of AI, the advances that shaped it, and how it powers modern tools like Watchdog, which uses AI for community moderation.
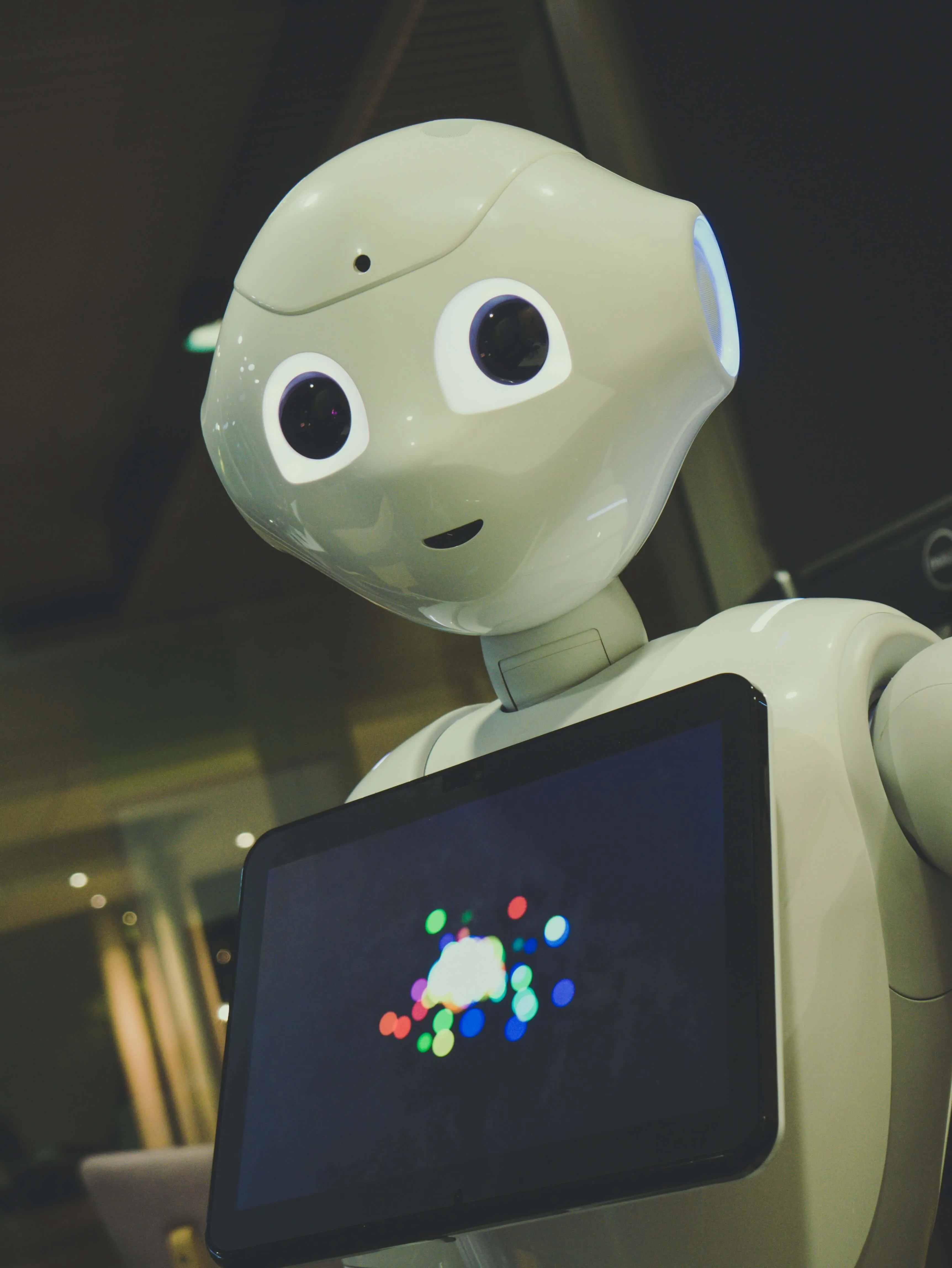
The Beginnings of AI: Theoretical Foundations in the 1940s
The inception of AI can be traced back to the 1940s, a period marked by advancements in mathematics and computer science. British mathematician Alan Turing is often credited as the “father of AI” due to his contributions to the theoretical underpinnings of machine intelligence. Turing’s work during WWII in cryptography led to the development of the Turing Test, a concept to evaluate a machine’s ability to exhibit human-like intelligence. According to the Turing Test, if a machine’s responses in a conversation are indistinguishable from those of a human, it can be considered “intelligent.”
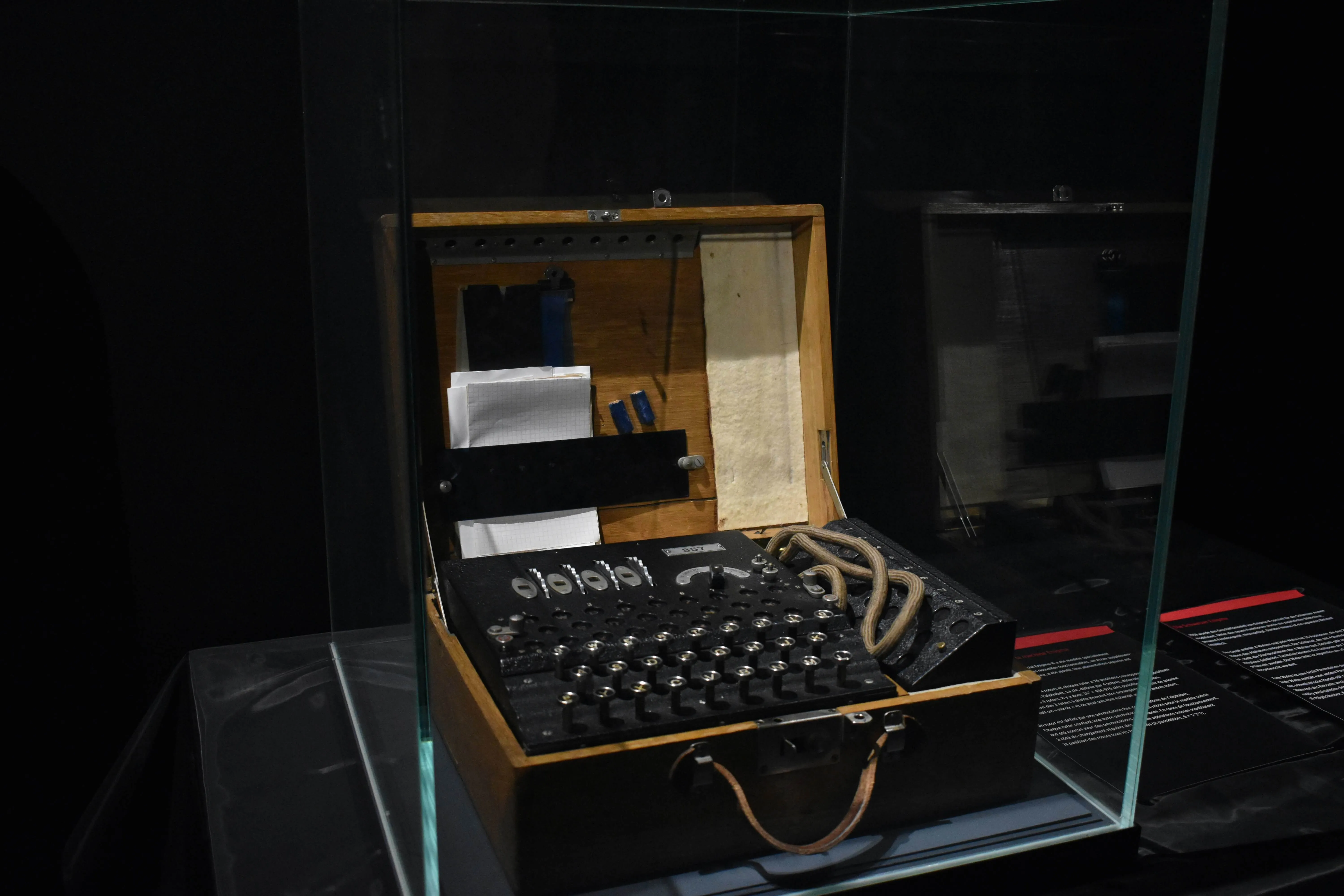
Turing’s ideas were groundbreaking, but they faced considerable skepticism. During this time, computers were primarily viewed as tools for computation rather than as entities capable of “thinking.” Despite the doubt, Turing’s vision planted a seed that would grow as technology advanced.
The First AI Program and Dartmouth Conference (1950s)
By the 1950s, AI began to shift from theory to experimental practice. In 1956, the Dartmouth Conference brought together pioneers like John McCarthy, Marvin Minsky, and Claude Shannon. These luminaries envisioned machines that could simulate human intelligence and even coined the term “artificial intelligence.”
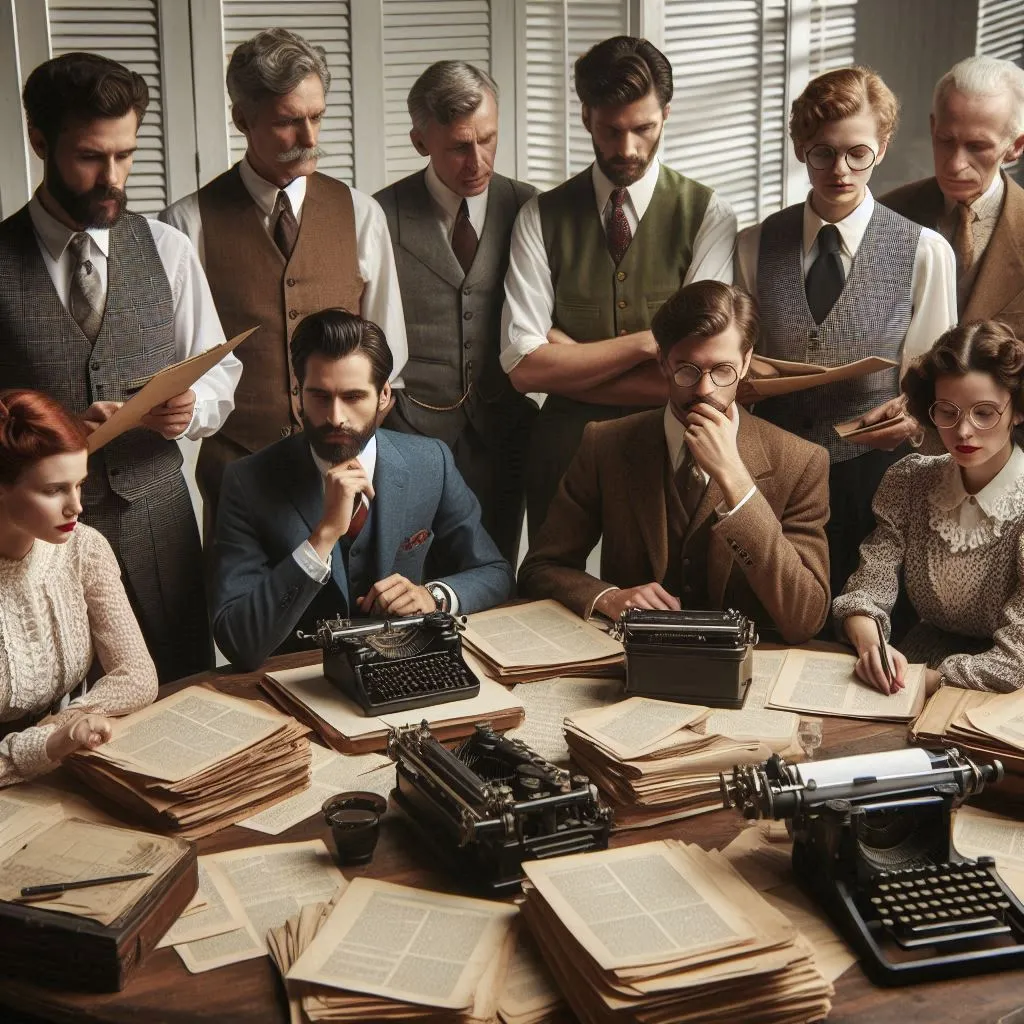
Following the conference, Logic Theorist, the first AI program, was created by Allen Newell and Herbert A. Simon. This program demonstrated the potential of machines to solve problems through logical reasoning by proving mathematical theorems. Logic Theorist marked a significant leap forward, proving that machines could, indeed, mimic certain aspects of human thought.
Key Breakthroughs in Early AI
This period saw the creation of programs capable of problem-solving and pattern recognition, albeit in limited capacities. AI pioneers believed that machines could achieve human-level intelligence within a few decades—a level of optimism that, unfortunately, would not withstand the realities of limited computational power and data access.
The Challenges of AI: The First “AI Winter” (1960s-1970s)
Despite the early enthusiasm, the AI field encountered significant obstacles in the 1960s and 70s. While researchers made strides in areas like natural language processing (NLP) and robotics, funding waned as advancements failed to meet expectations. This funding decline, known as the AI Winter, underscored the difficulty of making AI both powerful and efficient within the technological constraints of the time.
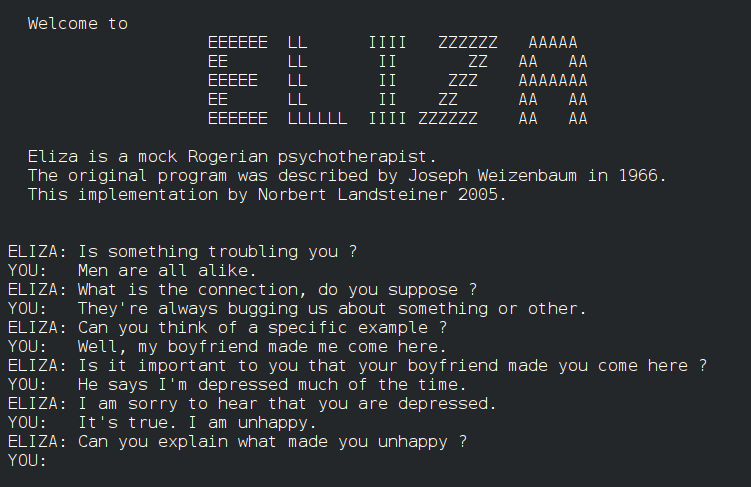
Notable, however, was the development of ELIZA, a primitive chatbot created by Joseph Weizenbaum. ELIZA simulated human conversation, marking an early attempt at interactive AI. While rudimentary by today’s standards, ELIZA demonstrated the potential of AI to engage with humans, a concept that remains central to technologies like chatbots and virtual assistants.
Renewed Interest in AI: The Rise of Expert Systems (1980s)
In the 1980s, AI saw a revival with the emergence of expert systems—programs designed to mimic the decision-making abilities of human experts. These systems found applications in specialized fields like medical diagnostics and finance, showcasing AI’s potential in practical applications. MYCIN, one of the earliest expert systems, assisted doctors by recommending antibiotic treatments based on medical data, highlighting how AI could extend human expertise.
With expert systems, AI shifted from broad aspirations to specialized tools designed for specific tasks. This era also saw significant advances in machine learning, paving the way for algorithms that could learn from data—a foundational concept for modern AI.
Breakthrough Moments: Deep Blue and Early Machine Learning (1990s)
The 1990s marked a turning point for AI. Improvements in computational power and data storage made AI more feasible for practical applications. IBM’s Deep Blue made headlines in 1997 when it defeated world chess champion Garry Kasparov. This victory demonstrated that machines could excel at tasks requiring strategic thinking, a hallmark of intelligence.
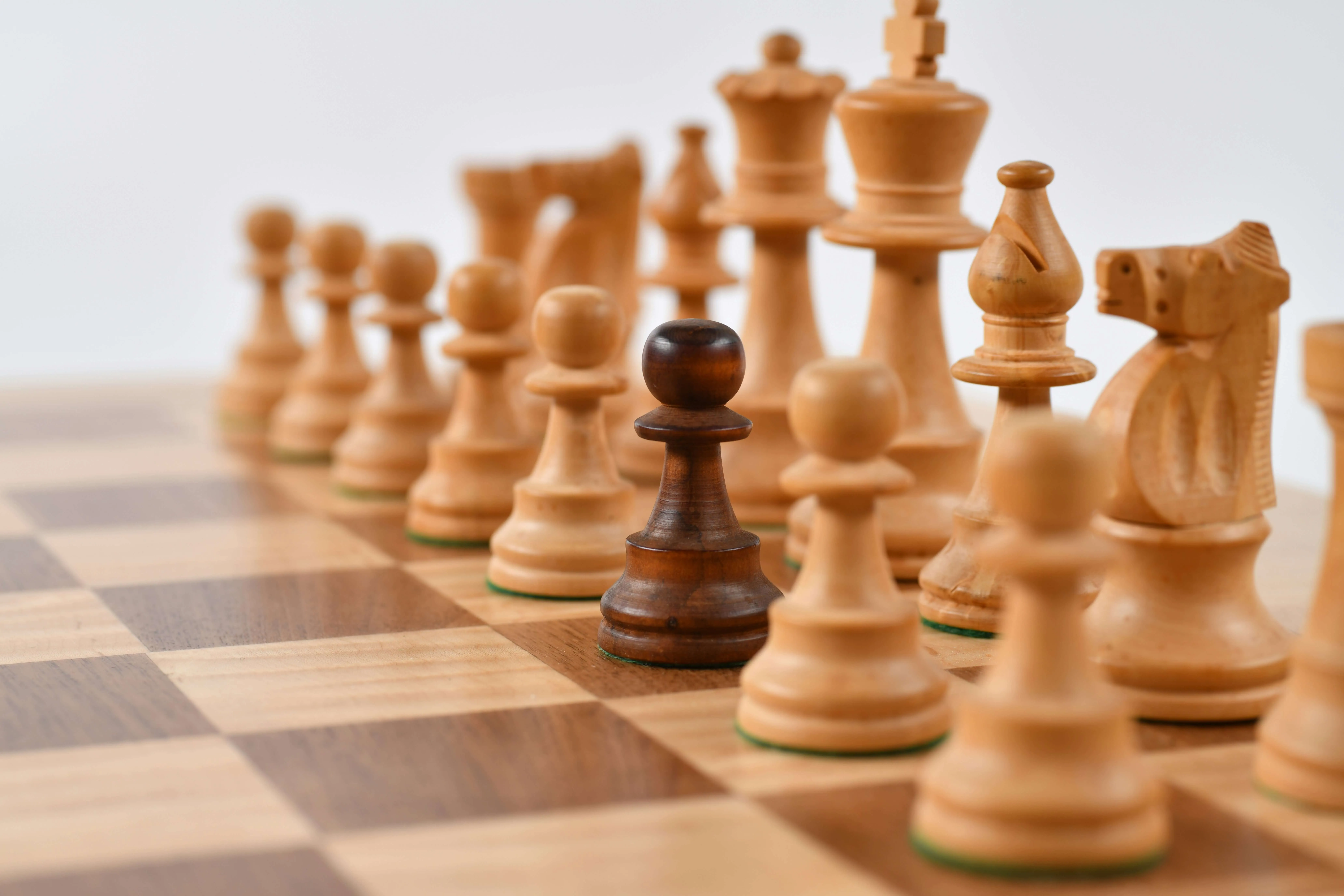
During this period, AI researchers also began to explore early forms of machine learning (ML), which enabled systems to improve their performance based on data rather than pre-programmed rules. These developments formed the basis for later advancements in natural language processing and computer vision, two key areas that underpin AI applications today.
The Explosion of AI: Big Data and Deep Learning (2000s-2010s)
The 2000s ushered in the era of big data and deep learning. With the rise of the internet, vast amounts of data became available, providing the fuel necessary for more complex machine learning models. At the same time, improvements in graphics processing units (GPUs) enabled researchers to train deep neural networks on large datasets.
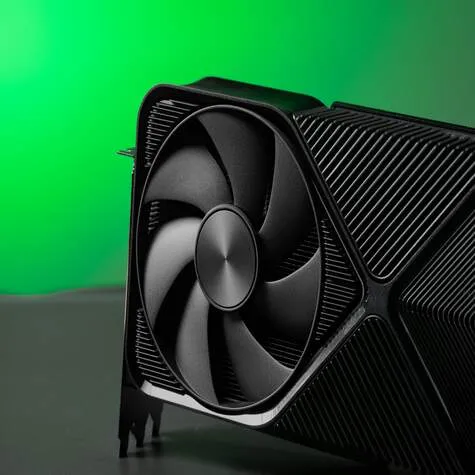
This period saw the birth of self-driving cars, facial recognition, and speech recognition—technologies made possible by deep learning algorithms. Virtual assistants like Siri and Alexa emerged, showcasing AI’s ability to interact with users in intuitive, conversational ways. AI had transformed from a niche technology to a ubiquitous tool.
Modern AI and Real-World Applications (2010s-Present)
Today, AI is part of nearly every industry. From healthcare to finance, AI systems analyze massive datasets to find patterns, make predictions, and automate decision-making processes. Natural language processing (NLP), computer vision, and reinforcement learning have reached levels that make AI capable of understanding and generating human-like text, recognizing images, and learning through trial and error.
One area where AI has seen substantial impact is community moderation. Online communities require constant oversight to ensure that interactions remain positive and rule-compliant. Platforms like Watchdog utilize AI to analyze messages in real-time, identifying potentially harmful or rule-violating content. By doing so, Watchdog empowers community moderators to maintain a safe and inclusive environment without the need for constant human intervention.
How Watchdog Uses AI for Community Moderation
Watchdog is an example of how far AI has come and how it can be applied to real-world challenges. Built with advanced natural language processing, Watchdog scans community messages for indicators of rule-breaking behavior, including harassment, inappropriate language, and solicitation.
Unlike traditional moderation, which relies heavily on human moderators, Watchdog’s AI analyzes language patterns and conversational context, allowing it to respond quickly and accurately to potential issues. This is particularly valuable for large communities where manual moderation would be time-intensive and potentially less effective.
Why AI Moderation Matters
AI-powered moderation tools like Watchdog are essential for digital communities. They help maintain a respectful and safe environment, which is critical for fostering engagement and trust. AI moderation tools also offer scalability, allowing growing communities to maintain quality interactions without requiring proportionate increases in human moderation resources.
The Future of AI: What Lies Ahead?
The future of AI holds exciting possibilities. Emerging technologies like quantum computing could further accelerate AI’s capabilities, making it feasible to process and analyze data at unprecedented speeds. Additionally, developments in explainable AI aim to make AI models more transparent, allowing users to understand why an AI makes certain decisions—a key feature for applications in healthcare, law, and finance.
For platforms like Watchdog, the future could bring even more sophisticated moderation capabilities. As AI models become better at understanding nuances in language, they may be able to detect not just rule violations but also potential misunderstandings or emotionally charged exchanges. This proactive approach could lead to more harmonious and engaged communities.
Conclusion: AI’s Journey from Idea to Reality
From Alan Turing’s early ideas to today’s complex machine learning algorithms, AI has come a long way. Each era of AI development has brought us closer to realizing machines capable of not only understanding human language but also responding in meaningful ways. This journey has created tools that help us in countless ways, from virtual assistants to automated moderation platforms like Watchdog.
By using AI, Watchdog provides community managers with a robust solution for moderating interactions, ensuring that online spaces remain safe and welcoming. As we look to the future, AI will undoubtedly continue to transform our lives, and tools like Watchdog will play a pivotal role in making that transformation positive and impactful.